Auto-MISCHBARES
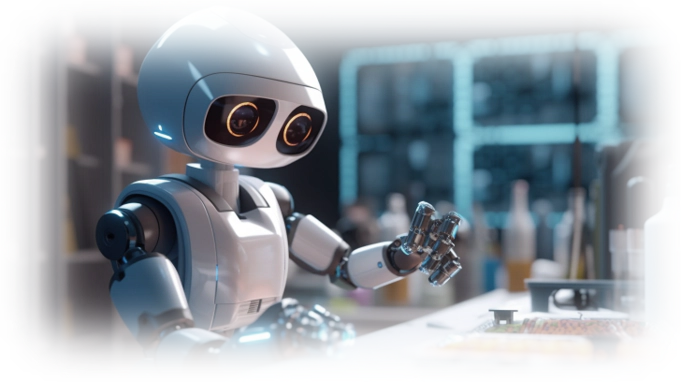
Auto-MISCHBARES, building upon our HELAO framework, is designed for high-throughput electrochemical research. It automates the study of different electrolyte and/or electrode materials, different electrochemical protocols in order to characterize the interphase formations at a millimeter scale, enhancing the efficiency of material discovery. This system’s significant feature is its ability to autonomously asynchronously orchestrate sequential or parallel experiments, integrated with advanced Quality Control assessments and MADAP for advanced data analysis using AI algorithms. The web interface of Auto-MISCHBARES offers streamlined user control, and its database design adheres to FAIR principles, promoting robust and transparent research in battery material science.