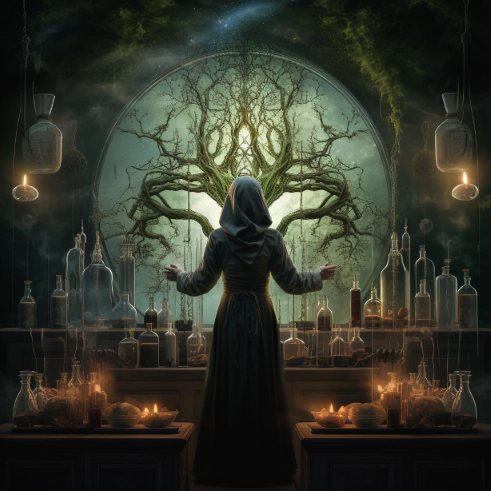
The model provides robust multi-task prediction capabilities through its modular design and the ability to dynamically adjust. It processes a wide range of historical battery cycle data to provide foundational insights for initial degradation detection. Incorporating predefined operational parameters, such as discharge rates and cycle numbers, ARCANA offers a dual analytical approach. This approach, coupled with the model’s inherent ability to include uncertainty in its predictions, presents ARCANA as an essential framework, which enhances the strategic development of battery maintenance protocols as well as reliability and longevity in energy storage applications.